Dec 23, 2024
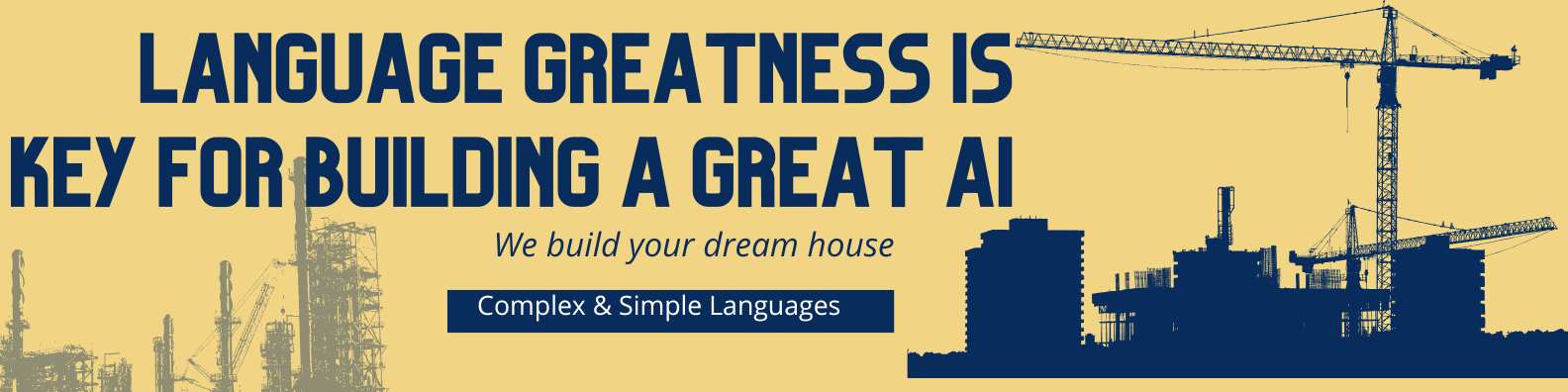
Language plays a key role in greatness of AI
The relationship between language complexity and information density in communication is a nuanced topic, touching on linguistics, cognitive science, and even social dynamics. Here we will discuss how we humans tended towards simpler languages for brevity and average mass acumen. And finally we discuss how that is impacting our progress towards achieving decent accuracy for AI (artificial intelligence) and AGI (artificial general intelligence). Below is a summary of key points which could define the language complexity and help us discover the larger topic of impact on evolution of AI technology :
1. Definition of Language Complexity
- Structural complexity: Refers to the grammar, syntax, and morphology of a language. Complex languages often have intricate rules, such as inflectional morphology or nuanced word order.
- Lexical complexity: Refers to the size and specificity of a language's vocabulary.
- Semantic complexity: Refers to how concepts are encoded and how much ambiguity exists.
2. Information Density
Information density in communication measures how much meaning is conveyed per unit of speech or writing. High-density communication efficiently conveys ideas with fewer words or symbols, while low-density communication requires more effort or redundancy.
3. Impact of Language Complexity
Positive Effects
- Precision: Complex languages often allow for greater precision. For example, agglutinative languages like Turkish can pack extensive information into single words.
- Richness of Expression: Structural and lexical complexity enable nuanced and context-sensitive communication.
- Error Recovery: Redundancy in complex languages can prevent loss of meaning if part of the message is misunderstood or lost.
Negative Effects
- Cognitive Load: Complex grammar or syntax can increase the mental effort required for both speakers and listeners, leading to potential misunderstandings or oversimplifications.
- Ambiguity: Overly complex expressions may introduce ambiguity, as subtle distinctions might be misinterpreted.
- Loss in Translation: Complex languages are harder to translate faithfully into simpler languages, leading to loss of nuance and detail.
4. Trade-offs and Efficiency
- Simpler Languages: Languages with streamlined grammar (e.g., Mandarin Chinese) often rely more on context and shared knowledge, which can lead to higher information density but also greater risk of miscommunication in less shared contexts.
- Complex Languages: These may ensure clarity within a specific framework but can be less efficient, as they require more processing time and effort.
5. Social and Practical Implications
- Cross-Cultural Communication: Differences in language complexity can lead to information loss when translating between languages, particularly in technical or legal contexts.
- Language Evolution: Over time, languages may simplify or grow more complex, often balancing the need for efficiency with cultural expression.
- Technology's Role: Tools like translation software often struggle with highly complex languages, which may result in reduced information density or misinterpretation.
The Darwinian Evolution of Language: From Complexity to Simplicity
Language, much like biological organisms, evolves over time, shaped by both environmental pressures and the needs of its users. The Darwinian perspective on language suggests that languages might have originated from simple, proto-linguistic sounds or syllables, evolving into increasingly complex systems. However, unlike biological evolution, which has a well-supported linear framework, the evolution of language does not follow a universally linear path. Instead, it oscillates, adapting to cultural, cognitive, and technological changes.
This later part examines a pivotal phase in this evolution: the historical peak of linguistic complexity—exemplified by languages like Sanskrit, Greek, and Latin—and the subsequent shift toward simpler, more utilitarian languages. This shift, while offering practical advantages, poses significant challenges for artificial intelligence (AI) development, where the loss of linguistic complexity and information density becomes a bottleneck.
1. Historical Linguistic Peaks: Complexity as Power
Sanskrit, Greek, and Latin: The Apex of Linguistic Sophistication
- Structural Depth: Languages like Sanskrit, Greek, and Latin were marked by rich morphological and syntactical structures. These languages could encode vast amounts of information in precise ways, using intricate case systems, verb conjugations, and syntactic rules.
- Expressive Power: The high information density of these languages allowed for nuanced expression, suitable for conveying complex philosophical, scientific, and literary ideas. For instance, Sanskrit's ability to represent nested and hierarchical concepts makes it a natural fit for algorithmic reasoning.
- Suitability for AI: Hypothetically, such languages would provide an ideal foundation for AI, as their logical structure and semantic richness align closely with the needs of computational systems.
2. The Simplification Trend: From Complexity to Utility
Drivers of Simplification
- Democratization of Language: As societies grew larger and more diverse, languages simplified to facilitate communication among broader populations with varying linguistic proficiencies. For example, modern English shed many of the inflectional complexities of Old English.
- Efficiency and Speed: Simpler languages, relying heavily on word order and context rather than morphological markers, cater to fast-paced, everyday communication.
- Globalization: The rise of "global languages" like English, with streamlined grammar and a focus on ease of learning, prioritized accessibility over depth.
Consequences of Simplification
- Loss of Precision: Simpler languages often lack the means to convey subtle distinctions without added context, leading to potential ambiguities.
- Reduction in Information Density: Ideas that could once be expressed in a single, information-rich word or phrase now require more verbose explanations.
- Challenges for AI: Modern languages' reliance on context and idiomatic expressions makes natural language processing (NLP) and machine learning systems struggle with ambiguity and nuance.
3. Language Evolution and AI: The Bottleneck of Simplicity
Impact of Linguistic Simplification on AI
- Ambiguity and Context Dependence: Simplified languages rely on shared context for meaning, a challenge for AI, which often lacks the cognitive ability to infer nuanced meanings.
- Loss of Structural Cues: The decline of inflections, cases, and conjugations deprives AI systems of explicit markers that aid in parsing and understanding sentences.
- Increased Cognitive Load for Machines: AI must compensate for the lack of linguistic density with advanced algorithms, often requiring extensive training data and computational power.
Learning from Historical Complexity
- Rediscovering Dense Languages: By revisiting languages like Sanskrit, Greek, and Latin, AI systems can learn from their logical structures and semantic richness. For example, Sanskrit's precise grammar aligns well with formal logic systems, providing a template for algorithmic reasoning.
- Hybrid Models: Combining elements of historical linguistic complexity with modern simplifications could enhance NLP systems, allowing for richer and more precise communication.
4. A New Lens on Language Evolution
Challenges to the Darwinian View
- Non-Linear Evolution: Unlike biological evolution, language evolution lacks a single, unidirectional path. The shift from complexity to simplicity suggests cyclical patterns influenced by sociocultural dynamics.
- Multiple Origins Hypothesis: Language evolution may not have a singular point of origin but could involve convergent evolution, with different languages developing unique complexities independently.
Implications for Linguistic Theory
- Language as an Adaptive Tool: The evolution of language reflects a balance between precision, usability, and accessibility, much like the adaptive traits of biological species.
- The Role of Technology: As technology influences communication, a potential "recomplexification" of language may emerge, driven by AI's need for unambiguous, information-rich inputs.
Conclusion: Toward a Linguistic Renaissance
The Darwinian view of language evolution highlights a fascinating journey from simple sounds to highly complex systems, followed by a modern trend of simplification. While this trajectory has made communication more accessible, it presents significant challenges for artificial intelligence, which thrives on the kind of structural richness found in historical languages.
To address this bottleneck, revisiting and learning from the complexity of languages like Sanskrit, Greek, and Latin could provide invaluable insights. These languages, with their unparalleled capacity for precision and information density, offer a roadmap for enhancing AI and even reimagining modern linguistic systems. By bridging the gap between historical sophistication and contemporary utility, we can create a future where language, humans, and machines evolve in harmony.
Best
Discover the best practices of building best product experience from millions of ready-made product graphs or build one yourself.

Intelligent
In-depth intelligence of products in the form of product stories help in achieving quality, automation and efficiency in new and existing product implementations.

Augmented
Improve and augment end to end product selection, development, integration, and operation with detailed information and AI copilots.

